I. Introduction
As we live in a data-driven world, it’s important to understand the credibility of numbers. One crucial component of data analysis is calculating margin of error. In summary, margin of error is a statistical representation of the likelihood of error in your sample data. This article aims to provide a comprehensive guide to calculating margin of error so researchers and pollsters can make accurate decisions. We’ll also discuss common mistakes to avoid, how to interpret margin of error, and why it’s important to understand the concept of confidence intervals.
II. A Step-by-Step Guide: How to Calculate Margin of Error
Calculating margin of error can seem daunting, but it’s actually a simple formula. You need to consider the sample size, the level of confidence, and the size of the population. Then, calculate the standard error, which is the estimate of the amount of random sampling error in the survey’s results. Divide the standard error by the square root of the sample size, and then multiply it with a z-score from a standard normal distribution table.
For a quick and easy breakdown, here’s the formula: Margin of Error = Z * σ / √n. We’ll explain each component below.
Sample Size: Your sample size represents the number of people in your sample group. A larger sample size decreases the margin of error. A rule of thumb is to have a sample size that is at least 30 or more.
Level of Confidence: The level of confidence is the probability of receiving a sample result within a certain range. Common percentages are 90%, 95%, and 99%.
Size of the Population: Your population size is the number of individuals your survey data represents. Sometimes, it’s difficult to ascertain the population size exactly, in this case, you can assume it to be an exponentially large number.
Standard Error: Standard error is critical because it measures the variability in the data. To find the standard error, divide the standard deviation of the population by the square root of the sample size. This adjusts the total variance of the sample so that it accurately reflects the variance of the population.
Z-Score: The z-score reflects how many standard deviations are within the sample result you received. Check the standard z-score table to find the correct score that corresponds with your level of confidence.
Combining these components within the formula results in your margin of error for the survey!
Here’s an example for context: Suppose we want to conduct a survey to find out the level of confidence people have in the government’s COVID-19 response out of a population of 5,000. We randomly select 400 people to take our survey. We want a 95% level of confidence, so our z-score is 1.96. The standard deviation of the population is 3.5%. Utilizing the formula, we can use the following steps:
Margin of Error = 1.96 * 3.5 / √400
Margin of Error = 1.96 * 0.035 / √400
Margin of Error = 1.96 * 0.035 / 20
Margin of Error = 0.0343 or 3.43%
Therefore, our margin of error would be 3.43%, so we can confidently say that with 95% certainty, the confidence level in the government’s COVID-19 response ranges from 46.57% to 53.43% in the population.
III. The Importance of Calculating Margin of Error
Calculation of margin of error is crucial in data analysis, surveys, and decision-making. Without margin of error, researchers cannot measure the precision of their results. This could lead to several problems. One such problem is the inability to generalize results accurately or to make correct business decisions – both of which could result in significant financial loss. By computing a reliable margin of error, researchers and pollsters can be more confident in their results and therefore make more accurate decisions.
Furthermore, the margin of error also communicates the precision of survey results, which is particularly important in the world of politics. Accurate polls lead to greater trust in democratic institutions, whereas unreliable polls can undermine public faith in institutions. Therefore, understanding margin of error has particular importance in contexts where its findings could sway public opinion or policy.
One of the most important aspects of data interpretation is knowing potential errors or biases in your study. This leads to our next topic on the potential consequences of not calculating margin of error.
IV. Top Tips for Reducing Margin of Error
No study is perfect, and therefore, there will always be a margin of error. However, through careful planning and execution, researchers and pollsters can achieve a smaller margin of error. Here are a few tips:
1. Increase sample size: The larger your sample size is, the lower your margin of error. Therefore, if feasible, it is necessary to recruit more members into your study.
2. Randomize selection: The selection process for your data must be random. This gives all members of the population an equal chance of selection.
3. Improve response rates: The survey’s response rate improves reliability and is an important factor in reducing bias.
4. Hire professionals: Professional pollsters, survey designers, or statisticians may prevent the type of mistakes that can add to your margin of error.
5. Pilot Test: Pretest your questionnaire on a small number of participants to identify any problems that may impede the final results. This will also assure that all respondents properly understand the questions being asked.
These steps can help to reduce error and increase the accuracy of your study, although it is impossible to completely eliminate the margin of error.
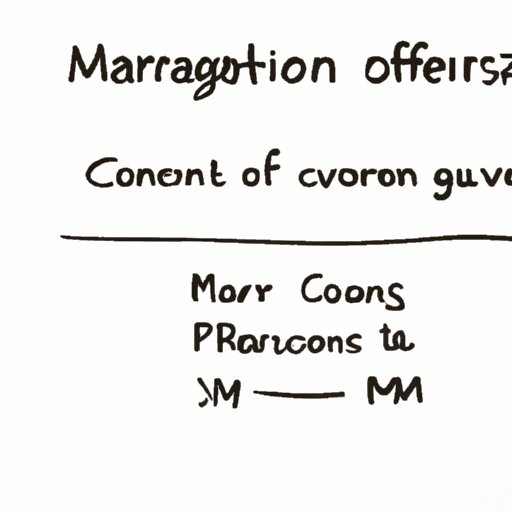
V. Common Mistakes to Avoid When Calculating Margin of Error
There are a few mistakes that researchers can make while computing margin of error that can have a substantial impact on results:
1. Using a small sample size
2. Choosing a low confidence level
3. Using inaccurate data while measuring standard error
4. Ignoring or miscalculating population size
5. Being unaware of the margin of error’s impact on the overall study
These mistakes reduce the reliability of your study and the validity of your result. Consequently, it is crucial to avoid these errors and to take further steps to understand how to calculate margin of error correctly.
VI. The Margin of Error vs. the Confidence Interval
It is vital to understand the difference between the margin of error and a confidence interval to interpret survey data effectively. A margin of error reflects the random sampling variability of the data. Conversely, a confidence interval quantifies the uncertainty about the true value of the parameter being estimated. A confidence interval can be indicated as “we are 95% confident that the actual difference is between _____ and ______.”
Therefore, while interpreting data, a confidence interval will give you a range of possible values for the parameter you want to estimate, and a margin of error will give you an estimate of the variability of your sample from the population. The relationship between the margin of error and confidence interval is due to these two values’ relation via a standard normal distribution table.
VII. How to Interpret Margin of Error
Interpreting the margin of error is crucial to good data analysis. In general, the margin of error provides a range of values that likely contains the true value of a statistic with a specified level of certainty. This level of certainty is usually indicated by a confidence interval, and researchers often indicate their certainty level in survey research, such as “with a 95% level of confidence.” Interpreting a good margin of error is essential and helpful to make accurate decisions based on survey data.
It is vital to note that if your margin of error is high, it implies the data is less accurate and more variable. Conversely, if your margin of error is low, your data is more precise and more indicative of the population’s larger picture. Therefore, researchers must control their study design and statistical analyses to minimize the margin of error while interpreting the margin of error in their constructed results.
VIII. Margin of Error in Real Life
Finding real-world applications where margin of error truly matters is not hard. One popular example is predicting presidential election results. A common method to measure voting preferences is by conducting a survey, and predictions may include a margin of error, anticipating that the candidate’s final result may fluctuate within the study’s range of data.
A crucial lesson is to consider the consequences of an incorrect margin of error. Suppose the margin of error is over 10%, and the pollster predicts a clear victory for one candidate. In that case, it is possible that this prediction might be wrong as the election leads to unexpected results. When evaluating different measures, researchers and pollsters must understand the margin of error impact to forecast accurate results.
IX. Conclusion
In conclusion, interpreting data requires a fundamental comprehension of margin of error’s concept and importance. Inaccurate margin of error can lead to several problems, so we explained a step-by-step methodology for researchers and pollsters to calculate it accurately. We discussed the importance of margin of error in surveys, data analysis, and decision-making. Additionally, we provided tips for reducing the margin of error, common mistakes to avoid, and the differences between margin of error and confidence interval. Through these discussions, we revealed the real-world relevance of margin of error. With this complete guide to margin of error, we hope that researchers and pollsters will accurately understand their data and utilize its full potential.